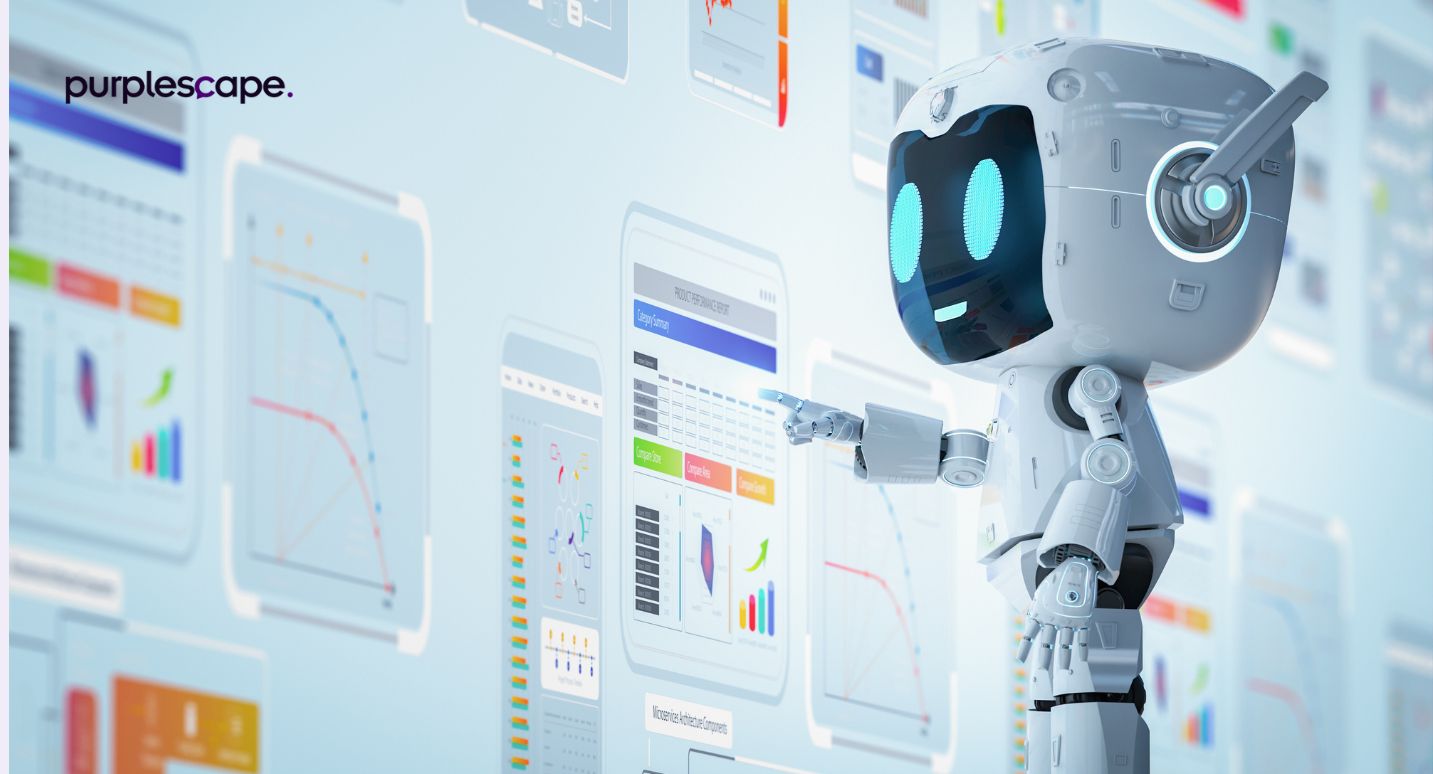
Introduction
Customer service has rapidly transformed in the last decade, driven by the rising expectations of consumers who demand quick, efficient, and personalized support. The traditional days of relying solely on human agents are long gone. Businesses today are integrating advanced technologies to handle interactions, resolve queries, and engage customers in ways that were once unimaginable.
Chatbots, once hailed as a revolutionary shift, became the face of this digital transformation, automating customer interactions and taking some pressure off human agents. However, technology continues to evolve, and chatbots, while useful, are no longer enough to meet modern customer demands. AI agents—highly intelligent systems that go beyond basic automation to deliver a proactive, human-like experience are starting to show their presence in the customer experience value chain. This blog explores the journey from basic chatbots to AI agents and how they are reshaping customer service today.
The Origins: Pre-Chatbot Era of Human-Centered Support
Before the introduction of chatbots, customer service was entirely human-centered. Customers had to interact with support teams through phone lines, emails, or face-to-face encounters. While this offered a personal touch, the limitations were clear—long wait times, inconsistent responses, and limited operational hours. The experience was often frustrating for customers, especially during high-demand periods when agents were overwhelmed by volumes of inquiries.
Early automation attempts included Interactive Voice Response (IVR) systems, designed to reduce the load on human agents by directing callers through automated menus. However, IVRs were limited and not very user-friendly. The system could only handle basic tasks like account inquiries, and more complex issues still required human intervention. This setup marked the beginning of technological assistance in customer service, but it was clear that better solutions were needed.
The Birth of Rule-Based Chatbots: Automating Simple Queries
The introduction of rule-based chatbots marked the next step in the evolution of customer service. These chatbots were designed to follow predefined scripts and were commonly deployed to manage simple, repetitive queries such as FAQs, order tracking, or business hours.
While these early chatbots played a vital role in reducing workload for customer service teams, they were limited by their rigid, rule-based design. They couldn’t handle conversations that veered outside of their pre-programmed responses. For example, if a customer had a query slightly more complex than what was in the chatbot’s database, the system would often fail, leaving customers frustrated and escalating calls to human agents.
This rudimentary system was helpful for businesses handling large volumes of low-priority requests, but it was not a replacement for skilled human interaction when it came to resolving nuanced or intricate issues.
Introduction to AI in CX: From Basic Chatbots to Conversational AI
The introduction of Natural Language Processing (NLP) and Machine Learning (ML) breathed new life into chatbot systems. No longer limited to scripted responses, chatbots evolved into conversational AI systems capable of interpreting human language and learning from interactions.
This shift allowed businesses to provide dynamic customer responses, enabling chatbots to engage in fluid conversations and understand intent beyond the basic query. NLP allowed chatbots to detect nuances in language, making interactions more natural and customer friendly.
For example, instead of simply providing store hours when a customer asked, conversational AI could recognize a follow-up query about product availability, suggesting options based on current stock levels. This elevated the role of AI-powered chatbots beyond mere task handlers into interactive, value-driven tools that could assist customers with more contextually relevant information.
Conversational AI Agents: Defining the Next Generation of Customer Interaction
As AI matured, the leap from chatbots to conversational AI agents became clear. AI agents are the next evolutionary step, equipped with advanced capabilities that set them apart from their chatbot predecessors. These AI agents can not only understand complex queries, but they can also learn from each interaction, improving their performance over time.
The key difference between chatbots and AI agents lies in their approach to context. AI agents are capable of understanding deeper context by analyzing the entire conversation history, not just individual queries. They can also integrate customer data, preferences, and behavior, allowing them to provide highly personalized and relevant responses.
AI agents don’t just answer queries—they engage in real-time decision-making and can autonomously resolve problems, such as processing refunds or managing order changes, without human intervention. This shift enables businesses to reduce reliance on human agents, allowing AI to take charge of more complex, time-sensitive customer interactions.
Continuous Learning: How Conversational AI Agents Improve Over Time
One of the most powerful features of AI agents is their ability to learn and adapt over time. Unlike static chatbots, AI agents improve their interactions based on machine learning models that analyze past customer conversations. They learn from mistakes, refine their responses, and become more accurate with each interaction.
For instance, if an AI agent frequently encounters similar queries, it will recognize patterns and adjust its responses to improve customer outcomes. Over time, this self-learning capability ensures that AI agents are equipped to handle even the most complex queries.
Additionally, AI agents can adapt to individual customer preferences, tailoring interactions to suit their communication style, preferred channels, and history of engagement with the business. This continuous improvement drives not only efficiency but also customer satisfaction, as interactions feel more personalized and responsive over time.
Beyond Chatbots: Conversational AI Agents and Proactive Customer Service
While traditional chatbots function reactively—responding to customer inquiries—AI agents are capable of proactive customer service. By analyzing previous customer interactions and related information, AI agents can anticipate potential issues and reach out to customers before problems escalate.
For example, if an AI agent notices that a customer’s subscription is about to expire, it can proactively offer renewal options. If it detects that a customer is struggling with a particular feature, it can initiate assistance without the customer having to ask for help.
This shift from reactive to proactive service means businesses can solve problems before customers even become aware of them, leading to higher satisfaction and brand loyalty.
Multi-Modal AI Agents: Integrating Voice, Text, and Visual Support
AI agents are not limited to one medium of communication. Unlike traditional chatbots that mainly interact via text, AI agents operate across various modes, including voice, text, and even visual support. This multimodal capability is a game-changer in customer service.
For example, AI-powered voice assistants can handle customer queries via phone calls, while visual AI tools can guide users through product setups or troubleshoot technical issues with step-by-step instructions having a DIY video embedded in each step. This flexibility allows businesses to meet customers where they are, offering support through their preferred communication channels.
Emotional Intelligence: AI That Understand Customer Sentiment
As Conversational AI agents continue to evolve, they are increasingly capable of understanding human emotions. Through advanced sentiment analysis, AI agents can detect whether a customer is frustrated, angry, or satisfied based on their language, tone, or behavior.
This ability to read emotions allows AI agents to adapt their responses to suit the customer’s emotional state. For instance, an AI agent can switch from a neutral, formal tone to a more empathetic one if it detects frustration. This kind of emotional intelligence helps build stronger customer relationships and enhances the overall service experience.
AI Agents in Crisis Management: Handling High-Stress Scenarios
AI agents excel in handling high-stress customer interactions, particularly during crises or urgent situations. In scenarios where human agents might become overwhelmed or emotionally charged, AI agents remain calm and logical, ensuring that customers receive clear and efficient solutions.
In high-stakes customer service environments—such as travel disruptions or booking cancellations— conversational AI agents can de-escalate situations by providing rapid yet empathetic responses with special curated offers that may help improve the overall brand image with a positive customer experience.
Conclusion
The evolution from chatbots to AI agents represents a transformative shift in customer service. Conversational AI agents are not only more capable than their chatbot predecessors, but they also offer a higher level of personalization, proactivity, and emotional intelligence. As businesses continue to adopt AI agents, the future of customer service looks set to become more efficient, human-like, and responsive than ever before. For business leaders, now is the time to explore how conversational AI agents can enhance customer interactions, improve operational efficiency, and foster deeper customer loyalty.