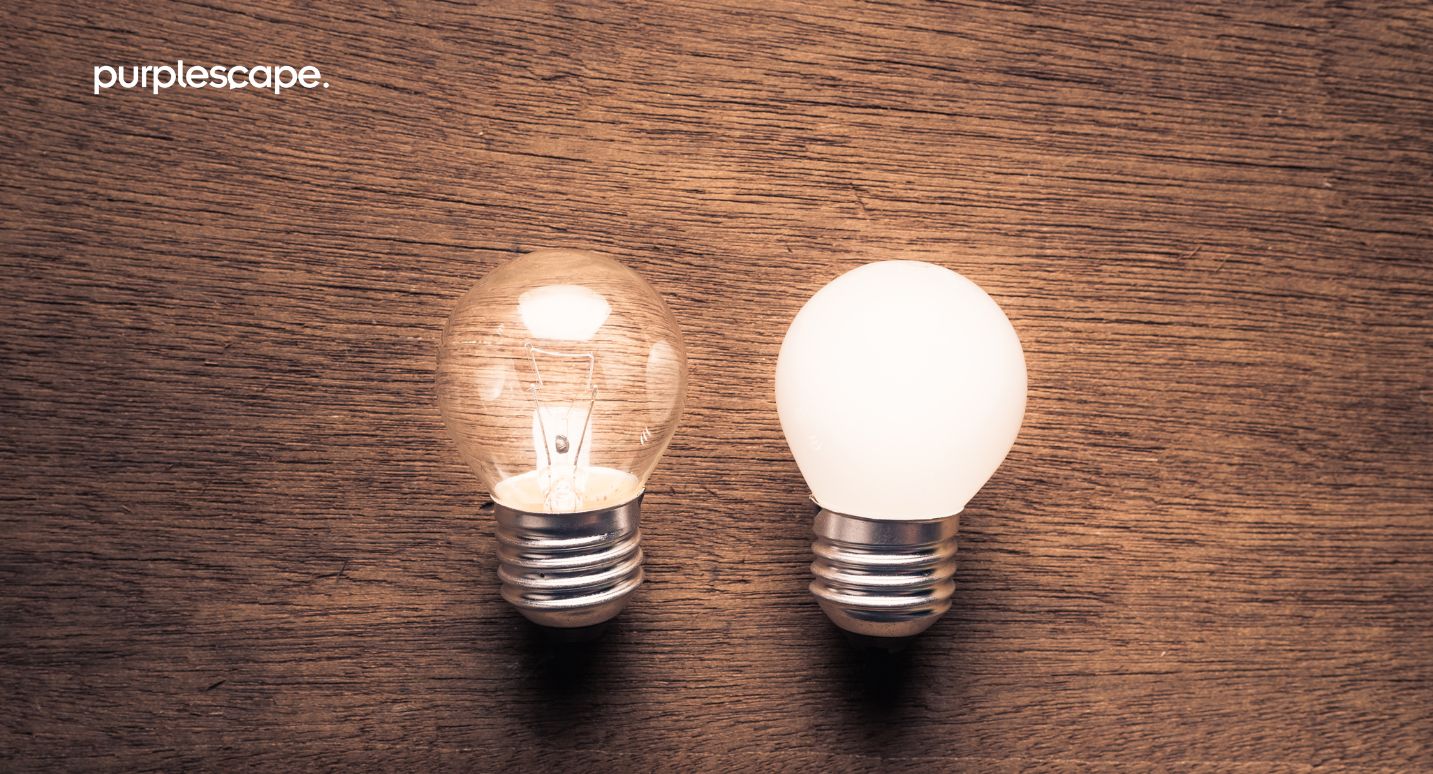
Introduction
Customer service stands at the forefront of the revolution to inculcate new technological advancements around the space of AI especially NLP, NLU, and NLG in their daily operations. AI systems have been integrated into customer support for over a decade, helping companies automate routine tasks and improve efficiency. However, with the emergence of Generative AI, a new phase in AI’s evolution is transforming how customer service operates. Understanding the key differences between Traditional AI and Generative AI is crucial for businesses looking to optimize their customer support.
This blog will explore the unique capabilities of both Traditional and Generative AI in customer service, diving into their historical evolution, differences in functionality, and their impact on business operations.
Historical Evolution of AI in Customer Service
AI’s role in customer service has evolved significantly over time, with key milestones marking the transition from rudimentary automation to the sophisticated, responsive systems of today. Understanding this progression provides valuable context for how Traditional AI and Generative AI fit into modern customer service strategies.
The Rise of Rule-Based AI Systems
AI made its first substantial impact on customer service with rule-based systems. In these early implementations, AI followed predefined scripts and decision trees to handle customer queries. These systems could answer basic questions but struggled with complex or nuanced issues. Companies used these systems to handle high volumes of routine requests like password resets or FAQs, freeing up human agents for more complicated cases.
Emergence of Traditional AI with Machine Learning
The introduction of machine learning into customer service marked the next stage of AI’s evolution. Traditional AI could now use algorithms to process data and “learn” from past interactions. This made the AI more flexible and capable of handling a broader range of queries. Businesses began deploying chatbots and virtual assistants that could improve over time, moving beyond static scripts to more dynamic, data-driven responses. These systems, however, were still limited to structured data and required continuous manual updates.
Conversational AI and Natural Language Understanding (NLU)
Advancements in NLU allowed AI systems to better understand and respond to customer inquiries. This led to the rise of conversational AI, which could interpret and process human language more naturally. While Traditional AI could now manage more complex queries, it still depended on structured datasets and predefined answers. It was a major improvement, but conversational AI was primarily task-oriented—focusing on automating specific processes rather than creating dynamic, human-like conversations.
The Introduction of Generative AI
Generative AI represented a significant leap forward. Unlike Traditional AI, Generative AI doesn’t rely on pre-existing scripts or strictly defined datasets. Instead, it uses large language models to generate real-time responses that are tailored to the context of the conversation. This breakthrough allowed businesses to handle more nuanced customer interactions and provided a more human-like experience, where the AI could respond to open-ended questions, generate creative solutions, and continuously improve from interactions.
Generative AI as a Customer Service Standard
Generative AI has been actively entering into the foray of customer service, especially for businesses aiming to provide seamless, personalized, and efficient customer engagement. With the ability to understand, learn, and generate complex responses, Generative AI is now capable of handling intricate customer problems, making recommendations, and even offering empathetic responses. Its adaptability and scalability have made it a key player in delivering modern customer support, far surpassing the capabilities of its traditional counterpart.
Capabilities: Rule-Based vs. Generative
When comparing Traditional AI to Generative AI in customer service, their core capabilities reveal distinct differences in how they approach tasks and solve problems. Traditional AI relies on predefined rules and is primarily suited for repetitive, structured tasks. On the other hand, Generative AI has the ability to create real-time, unique responses, making it a far more flexible and powerful tool for customer service. Below is a breakdown of how these two types of AI differ in their capabilities:
Aspect |
Traditional AI |
Generative AI |
Response Mechanism | Follows predefined scripts and workflows. | Generates dynamic, real-time responses. |
Flexibility | Limited to structured, repetitive tasks. | Handles unstructured tasks and adapts to new scenarios. |
Complexity Handling | Struggles with complex or multi-layered queries. | Excels in resolving complex and nuanced customer inquiries. |
Creativity | Cannot generate new content or ideas. | Can create new responses and solutions on the fly. |
Use of Data | Processes structured, labeled data only. | Leverages both structured and unstructured data to improve responses. |
Generative AI’s ability to go beyond pre-written scripts and learn from each interaction enables it to deliver a much richer, more personalized customer experience. In contrast, Traditional AI’s rule-based framework makes it effective for simple, repetitive tasks but less capable in handling dynamic and complex situations.
Data Utilization and Learning Models
Traditional AI operates on supervised learning, requiring human intervention to train the system with labeled data. While effective in many cases, this system depends on consistent updates and manual adjustments to remain relevant. If customer expectations change or a new product is introduced, Traditional AI systems need to be reprogrammed to address these shifts.
Generative AI, on the other hand, thrives on unsupervised and semi-supervised learning models. It continuously improves based on real-time data, extracting insights from every interaction and using those insights to enhance future responses. This ability to autonomously process and learn from data allows Generative AI to adapt rapidly without needing significant manual input, making it highly efficient for businesses.
Customization and Personalization
One of the most significant challenges for Traditional AI has been its limited ability to personalize interactions. While it can provide responses based on data inputs like customer history or previous interactions, the rigidity of its rule-based framework often results in generic responses. Even when a customer receives a personalized answer, it lacks the depth and dynamism needed to offer a truly tailored experience.
Generative AI, however, shines in personalization. By analyzing vast datasets in real time, including customer behavior, preferences, and interactions, Generative AI creates highly individualized responses that feel natural and relevant. The AI can go beyond scripted responses, dynamically adjusting its language, tone, and suggestions based on the customer’s unique profile and current needs.
Handling Complex Customer Interactions
When it comes to managing complex customer queries, Traditional AI’s limitations become evident. Traditional systems excel in handling simple, repetitive tasks but struggle with intricate or unpredictable problems. For example, if a customer issue spans multiple departments or requires a deep understanding of product details, Traditional AI systems are often unable to provide a satisfactory solution.
Generative AI, however, is purpose-built for these situations. Its ability to process unstructured data and generate dynamic responses allows it to tackle multifaceted issues. It can understand context better, ask relevant follow-up questions, and provide solutions that may not have been pre-programmed. This flexibility makes it especially effective in industries where customer queries can be highly variable and require more than just scripted answers.
Integration with Human Agents
Traditional AI, while efficient in many areas, often creates challenges when it needs to transition a customer to a human agent. Because these systems operate within rigid frameworks, they may not gather enough contextual information, forcing customers to repeat their issues when a human agent takes over. This disconnect can lead to frustration and inefficiency.
Generative AI eliminates this problem by capturing detailed contextual information during the interaction and passing it seamlessly to human agents. The AI system can summarize the customer’s inquiry, provide insights, and ensure that the agent is fully informed before they engage. This results in a smoother handoff, enhancing both customer satisfaction and agent efficiency.
Adaptability to New Customer Service Trends
One of the main drawbacks of Traditional AI is its slower adaptability to new trends. Traditional AI systems are highly dependent on structured data and predefined workflows, making it difficult to adjust quickly when new customer service trends or product lines emerge. This requires businesses to invest additional time and resources to manually update and reprogram the system.
Generative AI’s continuous learning capability means it can adapt to new customer expectations in real time. Whether it’s learning to handle inquiries about new products, adjusting to changing customer behaviors, or keeping up with emerging customer service trends, Generative AI evolves alongside the business. This adaptability makes it a powerful tool for companies looking to stay ahead of the curve in an ever-changing market.
Cost and Resource Implications
Traditional AI systems often require significant ongoing investments. While initial setup costs may be lower, the need for continuous updates, maintenance, and retraining of the system can lead to escalating operational expenses over time. This is particularly true for businesses that deal with a high volume of customer queries or where service offerings change frequently.
Generative AI, while it may have a higher upfront cost due to its complexity, offers significant savings over time. Its ability to learn autonomously reduces the need for manual intervention, making it more cost-effective in the long run. Moreover, the improvements in customer experience and satisfaction generated by AI’s personalized and dynamic capabilities offer a substantial return on investment.
Conclusion
The key differences between Traditional AI and Generative AI reveal the vast potential Generative AI holds for transforming customer service. While Traditional AI remains useful for automating simple tasks, Generative AI offers the adaptability, personalization, and complexity-handling that modern businesses need to meet the demands of today’s customers. As AI technology continues to evolve, businesses must assess their customer service goals and explore how Generative AI can offer a competitive advantage by delivering more efficient, personalized, and dynamic support.