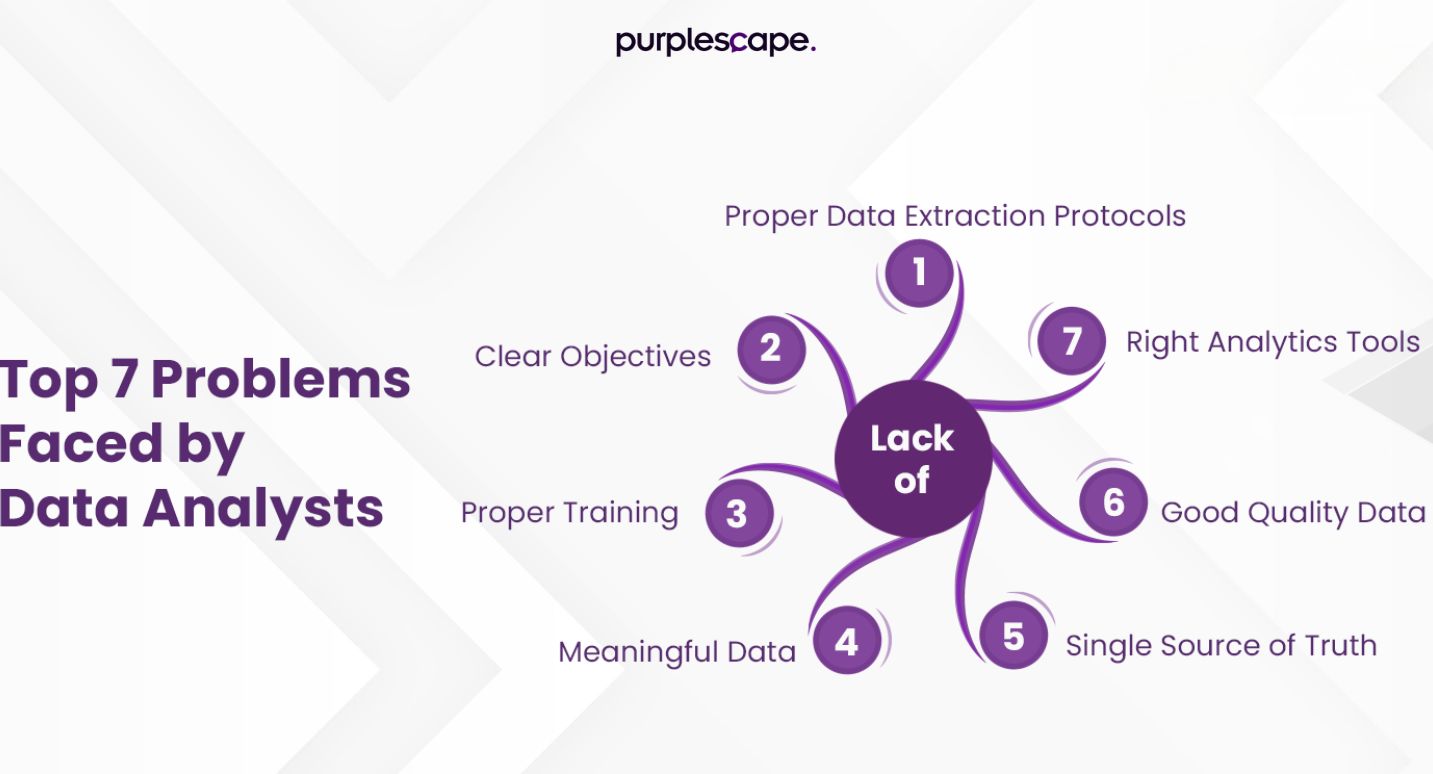
Introduction
Data analysts are a vital part of any organization. They ensure that the information provided by their company is used in the right way and that it has value. Data analysts must ensure top-end visibility exists for all their data projects ultimately ensuring the value they create with the data is noticed across the breadth and length of the organization. However, there are many problems faced by data analysts in their daily uphill battle with data.
The list is neither exhaustive nor is it a single-shot solution to the multitude of problems data analysts face. We have also consciously avoided issues like “data culture”, and “budgeting” for the simplest reason, it has been discussed time and again across articles. Also, they are beyond the control of the everyday analyst and may require a massive change at the organizational level. The problems discussed are according to some of the more pressing issues that require immediate resolutions and can be addressed without affecting a lot of organizational-level changes.
Lack of Clear Objectives
The first major problem that data analysts face is the lack of clarity on their objectives. If you don’t know what you are trying to achieve, then it becomes difficult for anyone else in your organization to help or support your efforts leading to confusion, frustration, and ultimately failure. So, every data analyst must start by setting the objectives for their data projects. Setting the objective is a result of multiple sessions with the business users across different functions to understand the end goal and curate data at each stage of the value chain.
Lack of Proper Training
It is essential for data analysts to have a basic understanding of data extraction, transformation, and loading. Data analysts should be trained in how to extract raw data from sources such as web pages or documents. They should also be trained on how to transform the raw data into useful formats before loading it into programs like Microsoft Excel or R Programming Language.
However, the explained skillsets are the bare minimum requirements for any data analyst. Given the speed at which the world of data analytics has evolved in the past few years, they must be at the top of their game by continuously learning and getting certified in select courses. There is a catch though. By the time data analysts finish their course, analytics would have further advanced. There’s one way to circumvent this catch-22 situation. That’s to look for easy-to-use data analytics tools equipped with conversational insights.
Lack of Meaningful Data
The volume of data that is being collected is huge at different touchpoints. The other end of the spectrum is the polar opposite. The visibility into granular data is still poor. All this workload of sifting through data to gain insights falls on the shoulders of data analysts and many times it becomes overwhelming. Add to that, the inability to execute this task in real time. The results are obvious. Businesses are unable to take timely data-driven decisions. The simplest alternative is to investigate a tool like Kea that can give momentary insights into all the data questions.
Lack of a “Single Source of Truth”
Just like today’s data volume is huge so are the channels from which data is getting loaded. A few examples would be the website, retailer’s intranet solution, CRM tools, etc for customer-related information. For the supply chain, it could be barcode details scanned at multiple touch points such as warehouses and ports, modes of transport used, wharfage costs paid, etc. The diversity in the type of data collected is also huge. However, all of them are stored at different data stores across different formats including but not limited to spreadsheets, data lakes, data warehouses, etc.
A data analyst trying to prepare a report for the sales team (for example), has to collect data from all the mentioned systems along with looking for data in the finance data stores. Given that it’s human to err, and unfortunately not many organizations are divine to forgive, data analysts face the brunt of wrong reports or misrepresented dashboards. A simple but sure shot way out of this predicament is bringing in a single point of storage from where analysts can extract the required information.
Lack of Proper Data Extraction Protocols
ETL (Extract Transform Load) is a critical part of data analytics. It’s used to extract data from multiple sources, transform it, and load it into a data warehouse. Incorrectly performing ETL can lead to incorrect results. Incorrect extraction may cause discrepancies in your reporting process as well as errors in reporting. Delays in reporting will occur if there are issues with loading or transformation processes. A way to address the issue is to automate data pipelines thus ensuring good data management processes. Automating data pipelines also ensures minimum human intervention is required along with reducing the common errors associated with general data management.
Lack of Good Quality Data
Bad data quality is one of the most common problems faced by data analysts. This can cause problems in analysis as well as hinder decision-making. Bad quality data is not a new phenomenon. In fact, it’s centuries old and can be attributed to the time humans started recording information. Even after introducing technologies to record, store, and analyze data, common issues like duplicate data (same customer names getting repeated twice), incomplete data (entering a mobile number without the area code), and inconsistent data (Entering the first name and last name for one customer, while not for the other) exist.
The issues surrounding data quality exist because the collected data is not passing through stringent quality checks. To ensure that error free data flow is available for analysis, tools inbuilt with proper data quality checks need to be implemented.
Lack of the Right Analytics Tools
The more data that you have, the better off your organization will be. However, as soon as you start collecting more information than ever before, it becomes essential for analysts to have access to the right tools for them to analyze their data effectively and efficiently. Without proper tools and methods at hand, analysts could end up wasting time on tasks that are not relevant or helpful in any way whatsoever.
Unfortunately, that’s currently where most data analysts spend their time. They end up with the clerical task of creating colorful dashboards and in-depth reports whereas they should be researching how the data inside an organization can add more value. The reasons are many but the major factor is that the modern suite of analytics tools is technically complex. Data analysts must be at the forefront to bring innovation into the world of data analytics. They should be the champions of easy-to-use tools with the most intuitive interface – Using language to derive insights.
Final Thoughts
Data analysts are under pressure to use data for a wide range of purposes. They should be able to communicate their findings effectively and they also need training on how to do this. The best way of solving the predicaments is by involving the data analyst in the design process so that they know exactly what they will be communicating through their data. Data analysts must have access to good tools and it’s important that these tools are easy-to-use, allowing information access without the need for too much technical knowledge. Therefore, it reduces the chances of making mistakes.
While some of the issues can be fixed by training and communication, others require either better tools or automation. There is no doubt that the role of a data analyst is important and will continue to grow in importance in the coming years. However, as the world looks for innovative ways to access insights, data analysts should also understand theirs will transition into a more value-added boundary role between the data and the organization. For that to happen, data analysts must be the voice of reason to introduce simpler ways to access information.