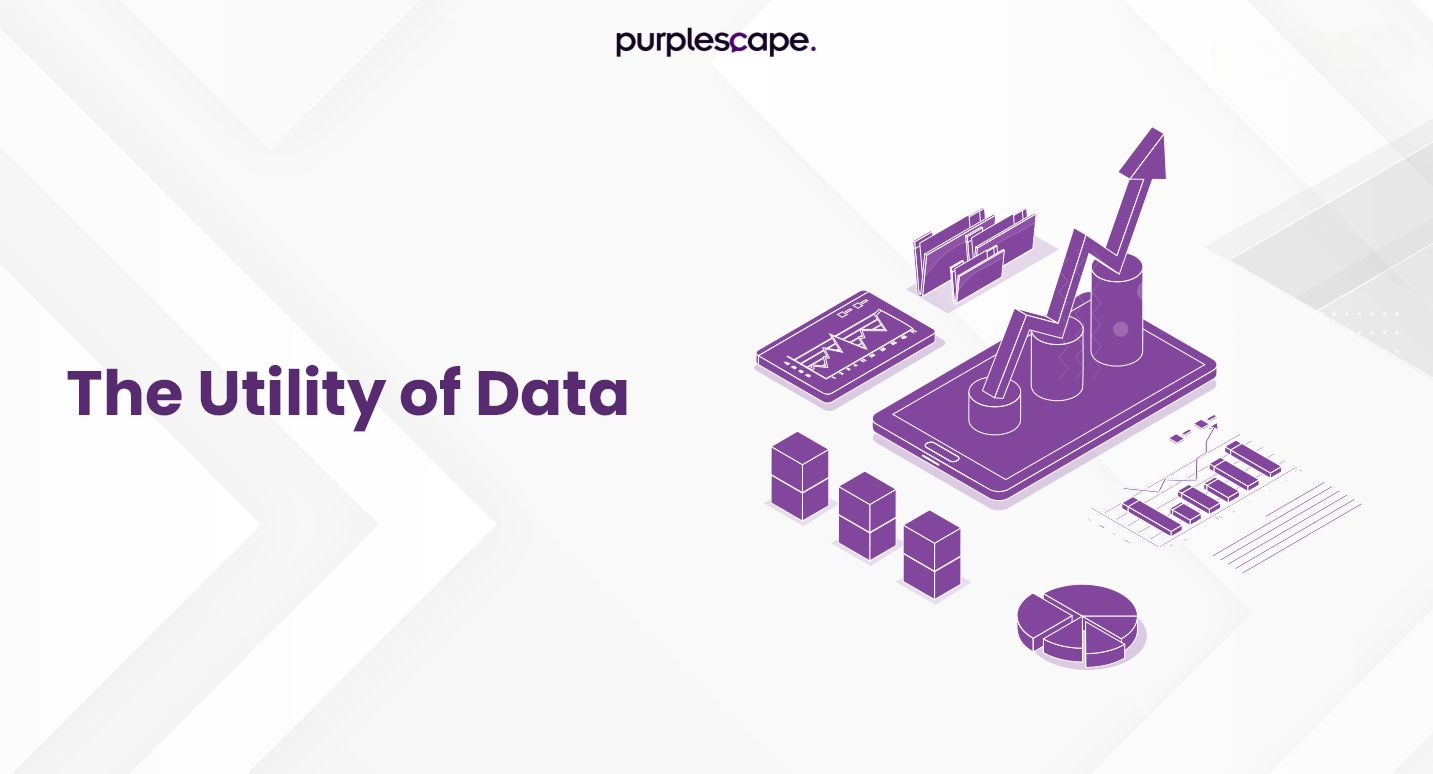
Data is the new oil. Data is the new soil. Data Scientist – the sexiest job title in the industry. AI First world, Augmented Analytics, Quantum reasoning, Analytics Engineering.
In what appears to be a primal need in staying informed, becoming knowledgable and perceive the world in an objective by effectively leveraging data, one of the biggest impediments I have seen coming in the way is the vagueness and intimidation of the terminology definitions and jargons that are used to drive home the message.
End of the day, all of them try to emphasize the point that a fact-based world is essential and why it is highly imperative to create one and how you can go about achieving that. Just like any modern-day cliche, the fundamental trap that many seem to fall prey into, is to put the cart before the horse and to get carried away by the fancy vernacular. Plenty has been written about Big Data, Small Data, BI, Data Science and most recently on AI that the need more often gets placed around being fashionable than being useful.
Let’s remember one fundamental ground rule. Digitization and hence data aid only with three things –
Observe, Infer and Act.
Observe – record a piece of information to know how anything would look at any given point in time. Or, right at this moment, based on how current the data is.
Infer – what do I learn from that observation? How do things relate to each other? How have things changed over time? What contributes to the observation? What would it look like in the future?
Act – What do I do next? Make some changes that in turn might affect future observations.
That’s it.
Nothing more and nothing less. Those three different objectives and the extent to which we are successful with those very much define the utility value of data – the reason why we would like to capture, store and consume.
The more you read about it, it might appear the term ‘data’ became fancy only in the recent past. Not that we have never used data for decision making or we have never been ‘data driven’ in the past. Civilisations and societies have relied on recording a piece of information way back in time, inventing newer ways to record and benefit from it. From the days of the tally sticks to Ishango bones, to early civilizations keeping detailed records of grain inventories, to the Greeks keeping a count of the people who could conscript to the army, to the recent years when the most common census data was widely used by countries to keep a tab on the number of people and the resources they have. Or, there is this most common use case of observing activities in our daily life – scientific or otherwise – and recording the outcome. As humans, we would like to observe the world or leave a mark for the future generation to observe.
The common underlying theme of all these efforts and the very genesis of data collection has been ‘to observe’. Observe what has happened at a point in time. The only change has been the way we collected this piece of information with the advent of computers and the exponential growth of highly sophisticated digital technologies over the past few decades or so which has resulted in an extremely large volume of diverse data being collected for the purpose of Observation; perhaps better.
The next logical step to observation is what we do with those observations – Infer. What do we learn from those observations? How are they related to each other? What do they convey? Is there anything of interest to learn from those observations? The inference was an art, especially the ability to locate the nugget of inference from a volume of data. The story of the British physician, Dr Snow to map the cholera outbreak in London is a classic e ample of spatial analysis in days when geo-location services did not exist.
But, human reasoning abilities have their own limitations and so did our ability to apply mathematical formulae and statistics to those observations. Add to it the volume and diversity of the observations which have grown tremendously over the years.
Enter digital technologies in the Inference process. Computers played and continue to play a big role in the inference or reasoning process with their ability to crunch a high volume of the observations for meaningful outcomes, extrapolations and forecasts. The computers also helped us locate meaningful signals over the noise, especially with the exponential growth of data (observations). Increasingly the big data problem is becoming that one of locating the needle (aka meaningful inference) in the haystack.
The utility value of Data ends with the most important one – to Act. As humans, we consume information and become more knowledgeable or optionally we take action for the better. Going back to the story of the cholera epidemic in London, the eventual outcome of Dr Snow mapping the observations, studying them and inferring a pattern lead the authorities to finally take action on the common water pump that appeared to be the source of the infection. Similar actions are common in any of the data applications of today – users consume information from various analytical tools and take actions or decisions.
These days even the process of Action has gone digital with varying levels of dependencies on humans, from facilitating actions to highly autonomous, self-healing machines. The self-driving cars, cruise missiles and rockets are all examples of highly autonomous computers using data observations to Infer and take Actions, independently. In addition to this, there are various examples of digital solutions that help humans to automate things, take actions and optimize processes – business or otherwise.
Bottomline, the underlying theme of the utility value of data has not changed over time – from the pre-historic times to the early days of computing to the small data era to the big data era and even in the coming days. It’s all about the 3 things Observe, Infer and Act and nothing more; nothing less.
What has changed is the way we have achieved those three aspects at increasing levels of sophistication, with and without the help of computing devices and how it might change in the future with the advent of Artificial Intelligence – all towards making life and things better.
That kinda sets the ground straight and simple for any technology solution in the data analytics domain – how easily does it enhance the utility value of data with Observe, Infer and Act.