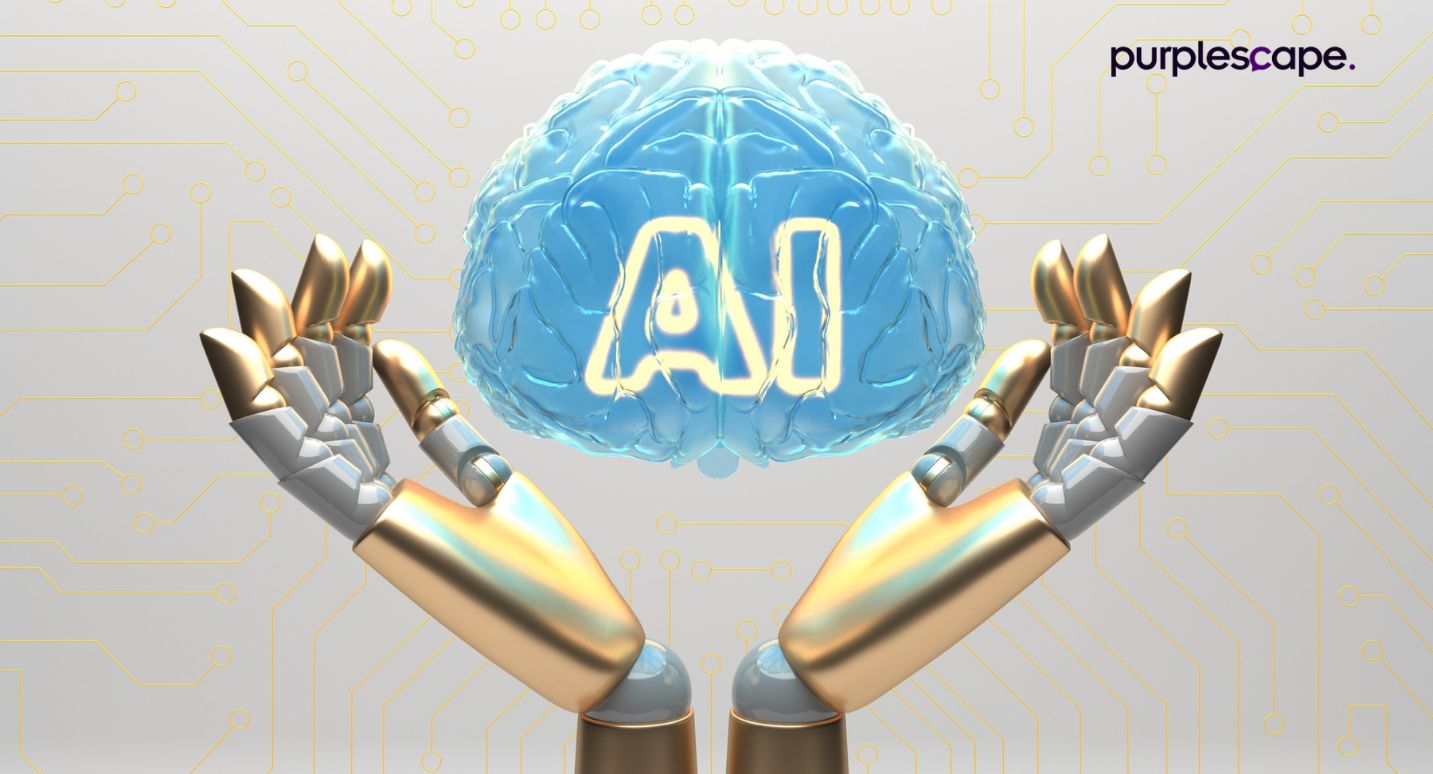
Introduction
Artificial Intelligence (AI) has long captured the imaginations of scientists, technologists, and futurists alike. Today, we are witnessing the emergence of a powerful subset of AI—Agentic AI—that is changing the landscape of industries across the globe. Unlike traditional AI, which requires substantial human oversight and control, Agentic AI represents a shift toward fully autonomous systems. These systems don’t just assist—they think, learn, adapt, and make decisions without human input, reshaping how we interact with technology, business, and even society at large. In this post, we’ll take you on a journey through the evolution of Agentic AI, explore its current applications, and envision its future potential.
1. The Pre-AI Era: The Dawn of Computing and Automation (Before 1950s)
Before the birth of artificial intelligence, the primary focus of technology was automation. Early computing machines, such as the ENIAC (Electronic Numerical Integrator and Computer), were designed for specific tasks—mostly calculation-heavy processes like military calculations and scientific problem-solving. These devices were important steppingstones in the technological revolution, but they were far from intelligent; they simply followed predefined instructions and did not exhibit any form of independent decision-making.
At this time, automation was primarily rule-based. Computers were programmed to perform repetitive tasks at incredible speeds, yet all decisions had to be directed by humans. The concept of intelligence, as we understand it today, didn’t exist—machines were tools, and the power of decision-making still lay squarely in human hands.
2. The Dawn of Artificial Intelligence: The Search for Intelligence (1950s – 1970s)
The 1950s to 1970s marked the initial attempts at replicating human intelligence. Visionaries like Alan Turing, who introduced the groundbreaking Turing Test in 1950, challenged the idea of machines being mere tools, asking whether they could think like humans. John McCarthy, often referred to as the father of AI, coined the term “Artificial Intelligence” and organized the first AI conference in 1956.
In these early days, AI systems were rule-based, designed to solve logical problems, play games like chess, and execute basic calculations. Despite the brilliance of these innovations, the systems were rigid, unable to learn or adapt. They could only perform tasks they were explicitly programmed to do—no matter how complex the tasks seemed to humans at the time.
This era of AI was largely focused on simulating human intelligence, but true autonomy—where machines could make decisions on their own—was still a distant dream.
3. Expert Systems: The First Step Toward Autonomy (1980s – 1990s)
As AI research progressed into the 1980s and 1990s, the focus shifted towards expert systems—programs designed to emulate the decision-making abilities of human experts in specific fields, like medicine, law, and finance. These systems were more advanced than their predecessors, capable of processing vast amounts of data and offering specialized solutions based on predefined rules.
While expert systems represented a significant leap forward in AI’s capabilities, they still depended heavily on human input. Their decisions were only as good as the rules they were given. They couldn’t learn from experience or adapt to new information, meaning their decision-making was limited to a fixed set of parameters. The concept of AI making independent, context-sensitive decisions was still far from reality.
Despite these limitations, expert systems were instrumental in laying the groundwork for what would later become Agentic AI—AI systems capable of not just performing tasks, but of evolving in response to changing environments.
4. The Rise of Machine Learning and Deep Learning: Laying the Foundations for Agentic AI (2000s)
The 2000s marked a pivotal period in AI evolution with the rise of machine learning (ML) and deep learning technologies. Unlike earlier AI systems that relied solely on human-designed rules, machine learning algorithms could learn from data, recognize patterns, and improve their performance over time. This marked a profound shift in AI’s capabilities—from mere automation to systems that could learn and adapt.
Deep learning, a subset of machine learning, took this a step further by mimicking how humans perceive the world. Machines could now recognize images, understand speech, process natural language, and even make predictions based on vast datasets. These breakthroughs were instrumental in moving AI systems closer to autonomy.
However, while these systems could make predictions and decisions based on data, they still required human supervision. The concept of an autonomous AI—one that could take action independently without human input—remained elusive.
5. The Emergence of Autonomous Systems: The Birth of Agentic AI (2010s)
The 2010s marked a turning point in the evolution of AI, ushering in the age of autonomous systems. With the advent of reinforcement learning and other advanced learning techniques, AI systems were now able to make decisions and take actions based on feedback from their environment, continuously improving without human guidance.
This was the beginning of Agentic AI—systems capable of making autonomous decisions, learning from their experiences, and adapting to dynamic environments. Technologies like self-driving cars, autonomous drones, and AI-powered robotics became reality. For example, autonomous vehicles used sensors and machine learning algorithms to navigate roads, avoid obstacles, and optimize driving patterns—all without human input.
These breakthroughs demonstrated that AI systems could not only assist humans but act independently, optimizing decisions in real-time based on an ever-evolving flow of data. This marked a leap forward in AI’s capabilities, with real-world applications that were previously thought to be out of reach.
6. The Current Era: Agentic AI in Business and Beyond (2020s)
Today, Agentic AI is no longer a futuristic concept; it has seamlessly integrated into industries, becoming a driving force behind real-time decision-making, process optimization, and the creation of entirely new business models. What was once seen as a far-off vision is now reshaping the fabric of industries such as healthcare, finance, retail, logistics, and manufacturing. These systems, equipped with the ability to learn, adapt, and make autonomous decisions, are changing the way organizations operate, reducing costs, improving efficiency, and unlocking innovative solutions that were previously unimaginable.
Healthcare
In the healthcare sector, Agentic AI is driving groundbreaking advancements in diagnostics, treatment planning, and patient care. AI algorithms now have the remarkable ability to analyze vast amounts of medical data—ranging from imaging and genetic data to patient histories—faster and with greater precision than ever before. For example, AI-powered systems like IBM Watson Health and Google DeepMind have demonstrated their capabilities in diagnosing complex diseases such as cancer and retinal conditions, achieving accuracy levels that rival seasoned healthcare professionals. In the operating room, autonomous robots are enhancing surgical precision by performing intricate movements with minimal human involvement, reducing the likelihood of errors and significantly improving patient outcomes.
BFSI
In finance, Agentic AI is reshaping the entire landscape, from algorithmic trading to fraud detection. AI models are adept at processing massive datasets in real-time, swiftly identifying patterns, and making decisions that can influence financial markets within moments. Within banks and financial institutions, AI-driven systems are revolutionizing risk assessment, credit scoring, and personalized financial advisory services—reducing the need for manual oversight. In some instances, these systems can autonomously execute trades or make financial decisions, not only improving operational efficiency but also reducing risks by minimizing human error.
Retail
In the retail sector, Agentic AI is transforming the customer experience and optimizing supply chain management. E-commerce leaders like Amazon leverage AI to dynamically adjust pricing, recommend personalized products, and manage inventory based on real-time consumer demand. AI-powered chatbots and virtual assistants provide customers with highly personalized shopping experiences, while advanced predictive analytics ensure that products are efficiently stocked and delivered with minimal human intervention. Additionally, AI’s ability to optimize logistics has resulted in faster delivery times, more streamlined warehouse management, and even the anticipation of future consumer demand.
Logistics
The logistics industry is also reaping the benefits of Agentic AI, particularly with the rise of autonomous vehicles and drones. AI-driven systems are being deployed to optimize delivery routes, reduce fuel consumption, and manage fleets of self-driving trucks with remarkable efficiency. Autonomous drones are revolutionizing last-mile delivery, especially in urban environments, offering fast, cost-effective delivery solutions. Furthermore, AI is enhancing warehouse automation, where robots autonomously handle tasks like picking, packing, and shipping products, driving substantial improvements in operational efficiency.
Software
In the software development space, the impact of Agentic AI is profound, particularly in areas like software testing and debugging. AI-powered tools now autonomously identify bugs in code, automate repetitive testing processes, and even generate new code based on predefined patterns. This innovation accelerates development cycles, enhances code quality, and enables faster deployment of software products. On the cybersecurity front, Agentic AI plays a critical role in enhancing the security infrastructure of modern organizations. AI-driven systems are continuously monitoring networks, identifying vulnerabilities, and responding autonomously to emerging threats—bolstering security and reducing the risk of human oversight.