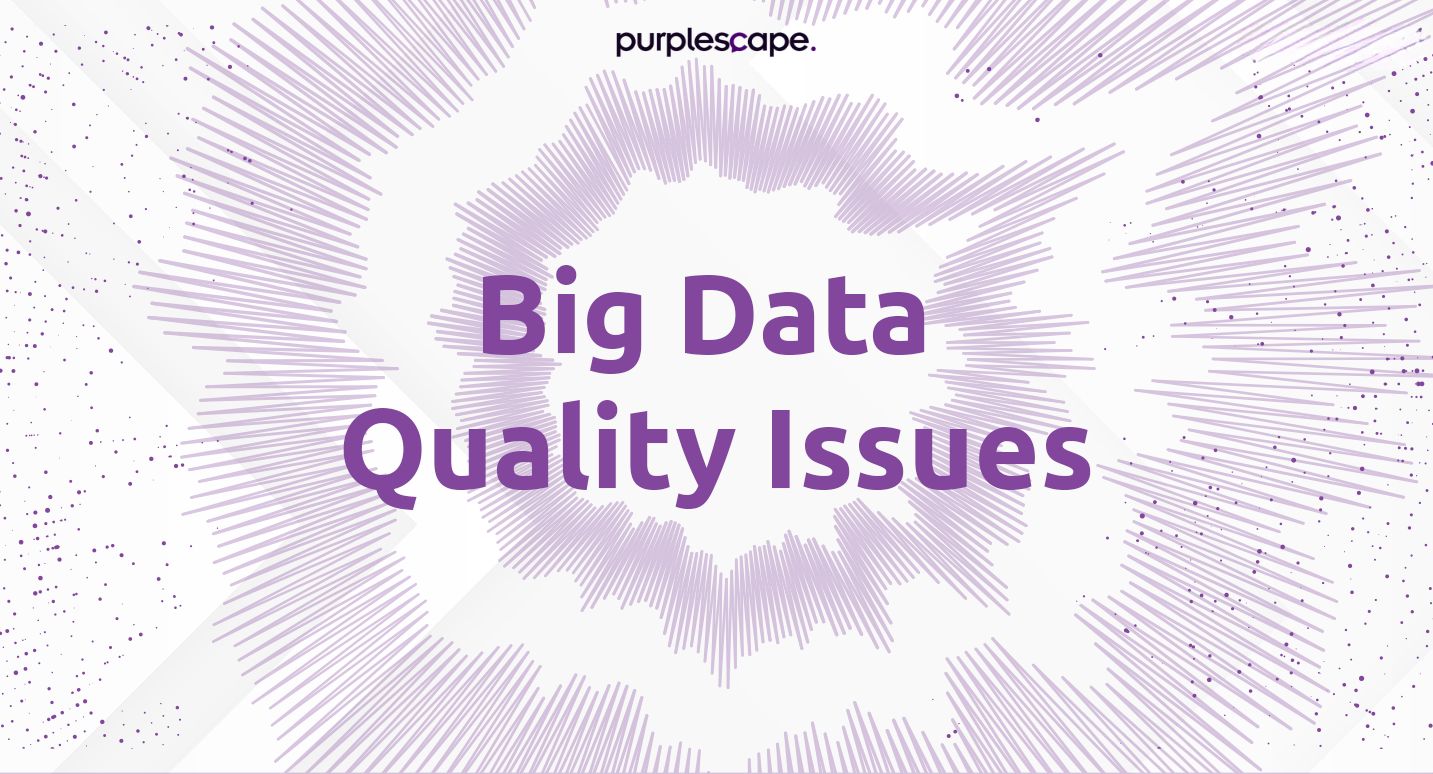
Introduction
Big Data quality issues have adverse consequences for any business, ranging from delegitimizing market campaigns and poor customer relations to negatively impacting decision-making. You might also experience stressful situations due to big data quality issues. However, handling inconsistencies and flaws in the data can enhance data analysis abilities and lead to better decision-making.
A minimal data quality issue can pull down your business, even for a short period. For this reason, we must look at some common significant data quality issues. Also, it is crucial to know how you can address these issues. Nevertheless, let us first look at the definition of data quality issues and why your data might have problems.
How Can We Define Big Data Quality Issues?
Big Data quality issues can be described as having an insufferable defect in the database. In this case, the fault diminishes the data’s trustworthiness and reliability. Data is an essential driver of your business functions. Therefore, with quality issues on your dataset, you may experience severe damage and risks in your entity.
How Do Big Data Quality Issues Occur?
As time goes by, datasets develop various issues. Unfortunately, quality issues are bound to occur with information stored in multiple sources. In most cases, many issues arise during the collection and data entry procedures. These problems result from individuals conducting the data entry or data collection systems.
Changes in customer information or formatting requirements over time also affect current datasets. Nevertheless, by having the right tools, data management, and entry plans, you can handle and correct any business issues that might emerge.
How to Address Big Data Quality Issues
Various common issues may affect data quality, from data collection mistakes to outdated information. Significant big data quality issues might be inevitable. However, they can be prevented. Therefore, it becomes essential to understand the common problems and devise ways to handle them. Here are the common issues that often arise during data collection and maintenance in your business.
1. Software Enabled Data Correction to Address Incomplete Data
Data incompleteness is where the necessary fields are absent in the database. Usually, during data entry, it is easy to overlook or intentionally fail to fill in some of the required fields in a form. Where there are several blank fields, you will most likely have big data quality issues with many records. This may affect the accuracy of conclusions you will draw from the data.
The good news is that an incomplete data issue is easy to fix through software that sets the necessary fields. When you have this kind of software, there are no forms that will be submitted without filling in the required fields. You can also opt to add rules to the questions and papers. Such restrictions may include permitting digits only in dates and currencies, depending on the information you need. Using either rules or the software, you will enhance data quality even before it enters the database.
2. Formatting Tools to Address Inconsistency
Numbers, addresses, and dates may result in formatting problems that make extensive data unhelpful and useless. When manually inputting information like dates, any number format is applicable: four-digit years, two-digit years, one-digit months and days, two-digit months and days, or a combination of each separated by slashes, hyphens, or spaces. In some situations, one might spell out the dates like “February 22nd, 2019”, subject to non-conformity and misspellings.
Numbers may not be as complicated as dates. However, they tend to fall into the same traps. Sometimes people may use “I” in place of “1” or an “O” in place of “0”. On the other hand, addresses are also impacted. This is where entries place the zip code in different address areas.
Formatting inconsistency negatively impacts the ability to analyze, compare data, and run reports effectively. Therefore, you need to assess and clean data regularly. With tools such as address validation tools, you can target and correct formatting issues. This will give room for consistency and improved data analysis.
3. Empowering Data Analysts with Tech to Combat Human Error
In data entry processes, human error is a natural component and a common challenge in big data quality issues. It may not be anyone’s fault, but it is a critical issue. Technology is an essential aspect of minimizing human error impacts. Nevertheless, people also play an important part in the process. Often, inputting data in the wrong fields and typos are common human errors. Others may include overlooking the necessary fields and intentionally giving incorrect information when submitting the forms. Though these mistakes are bound to happen, you can employ measures to correct or reduce them.
For any plan of data collection, training is a crucial element. However, offering proper training to data entry personnel may not be sufficient as they might make mistakes too. For this reason, cleansing and data validation technologies are the best options for identifying and flagging possible errors. Acquire the right data entry tools and you will never experience poor data quality from your datasets.
4. Deduplicating Data with the Right Product
Data duplication occurs when similar data is stored multiple times in the database. In most cases, replication may be unavoidable when using numerous methods and systems to collect data. You may get inaccurate results when you overlook duplicated information. Therefore, you need to have a system that often identifies duplicate data in the dataset.
Manually fixing duplicate data issues may be somewhat impossible. Furthermore, with the large amounts of data intake, addressing the same data may consume too much time. Therefore, you must invest in tools that combine and cleanse duplicated information to avoid duplicated data.
Final Thoughts
At this point, you now understand that big data quality issues are bound to happen when you frequently collect new data from multiple sources. Luckily, there are various resources at your disposal that can help in gathering and management of data. Whether you want to cleanse data already stored in the database or avoid data entry mistakes, various tools can help. Invest in the multiple tools available today and address big data quality issues.