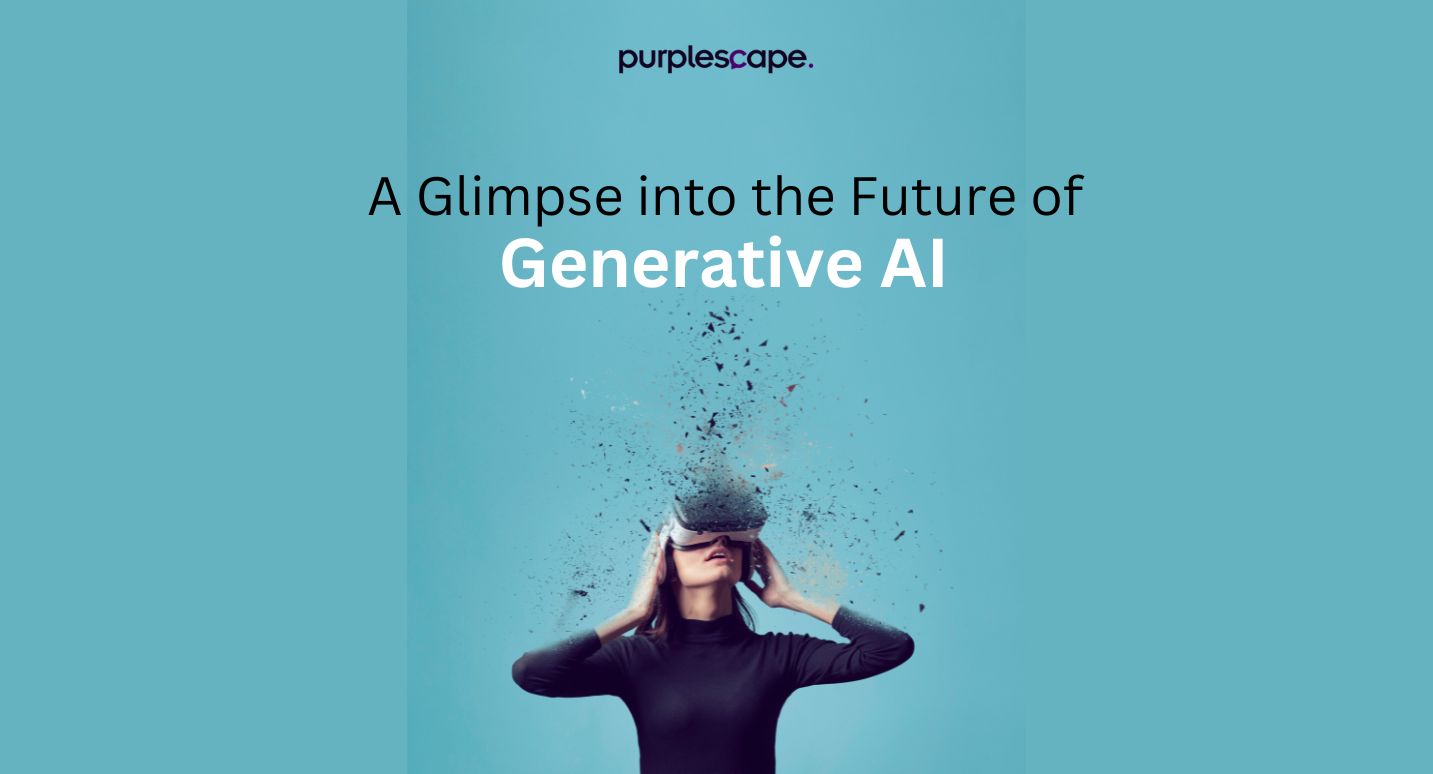
Introduction
We’ve automated text with Generative AI. We’ve automated voice with Generative AI. We’ve automated art with Generative AI. What’s the next step? Stitching them together into one ecosystem. This cutting-edge technology combines the power of multiple data modalities, such as text, images, and even audio, to create a more comprehensive and nuanced understanding of the world. As we delve into the intricacies of how multimodal generative AI works, and explore its industrial use cases across various functions, it becomes evident that this technology is poised to redefine the future of AI applications.
Understanding Multimodal Generative AI
Multimodal generative AI is an advanced form of artificial intelligence that goes beyond the limitations of single-modality models. Traditional AI systems often focused on processing and generating content within a single modality, such as generating text or images. However, the world we live in is inherently multimodal, with information conveyed through a combination of text, images, and sound.
Multimodal generative AI, like OpenAI’s CLIP and DALL-E, is designed to bridge these modalities, enabling machines to understand and generate content that encompasses the richness of human perception. The key to its functionality lies in the training process, where the model is exposed to diverse datasets containing examples from different modalities, allowing it to learn the intricate relationships between them.
How Multimodal Generative AI Works?
- Training with Diverse Datasets: Multimodal generative AI undergoes extensive training on datasets that include a mix of text, images, and audio. This training process helps the model develop a nuanced understanding of the relationships between these modalities.
- Learning Cross-Modal Representations: The model learns to create cross-modal representations, meaning it understands the connections between text, images, and audio. For example, it can learn that a particular image corresponds to a specific description in text or is associated with a certain sound.
- Generating Multimodal Content: Once trained, the multimodal generative AI can generate content that spans multiple modalities. This could involve generating a textual description of an image, creating an image based on a textual prompt, or even generating accompanying audio for a given visual scene.
- Fine-Tuning and Refinement: The model can be fine-tuned for specific tasks or domains to enhance its performance in particular applications. This adaptability makes it a versatile tool across various industries.
How can Multimodal Generative AI help industries?
- Content Creation and Design: Multimodal generative AI has immense potential in content creation and design. For instance, it can be employed to generate artistic visuals based on textual descriptions, design marketing materials, or even assist in the creation of multimedia presentations by understanding and combining text, images, and audio.
- E-Commerce and Visual Search: In the realm of e-commerce, multimodal generative AI can revolutionize the visual search experience. By understanding both textual queries and image content, it can provide more accurate and context-aware search results. This can enhance the user experience and drive better product recommendations.
- Healthcare Diagnostics: In healthcare, multimodal AI can be a game-changer for diagnostics. By analyzing medical images, textual patient records, and even voice notes from healthcare professionals, the AI can assist in identifying patterns and making more accurate diagnostic predictions.
- Autonomous Vehicles: The automotive industry stands to benefit significantly from multimodal generative AI. Vehicles equipped with AI systems that understand both visual data from cameras and textual or auditory instructions can navigate more safely and effectively. This technology contributes to the development of advanced driver-assistance systems (ADAS) and autonomous driving capabilities.
- Customer Service and Chatbots: Multimodal generative AI can enhance customer service by understanding and responding to both text and visual inputs. Chatbots, for instance, can better comprehend user queries by considering accompanying images or screenshots, providing more accurate and context-aware responses.
- Education and Training: In the field of education, multimodal generative AI can transform learning experience. It can generate educational content that combines text, images, and audio, catering to diverse learning styles. Additionally, it can be used to create interactive simulations and training materials for various professions.
- Entertainment and Gaming: The entertainment industry can leverage multimodal generative AI to create immersive experiences. Video games, for example, can incorporate AI-generated visuals, audio, and narratives that respond dynamically to user actions, leading to more engaging and personalized gaming experiences.
Parting Notes
As we stand at the intersection of various technological advancements, multimodal generative AI emerges as a beacon of innovation, promising a future where machines understand and interact with the world in a manner more akin to human perception. Its ability to seamlessly integrate text, images, and audio opens a multitude of possibilities across diverse industries, from healthcare and e-commerce to education and entertainment.
While technology is still evolving, the strides made in multimodal generative AI signal a paradigm shift in how we harness artificial intelligence. The transformative impact on industries underscores the potential for this technology to redefine the way we work, learn, and communicate. As research and development in multimodal generative AI continue to advance, we can anticipate a future where machines not only understand our world but actively contribute to its evolution in ways previously unimaginable.
Intrigued to know more about Generative AI? Check our blog on how ParrotGPT – Our Generative AI-powered conversational bot helps businesses achieve their short-term and long-term goals.