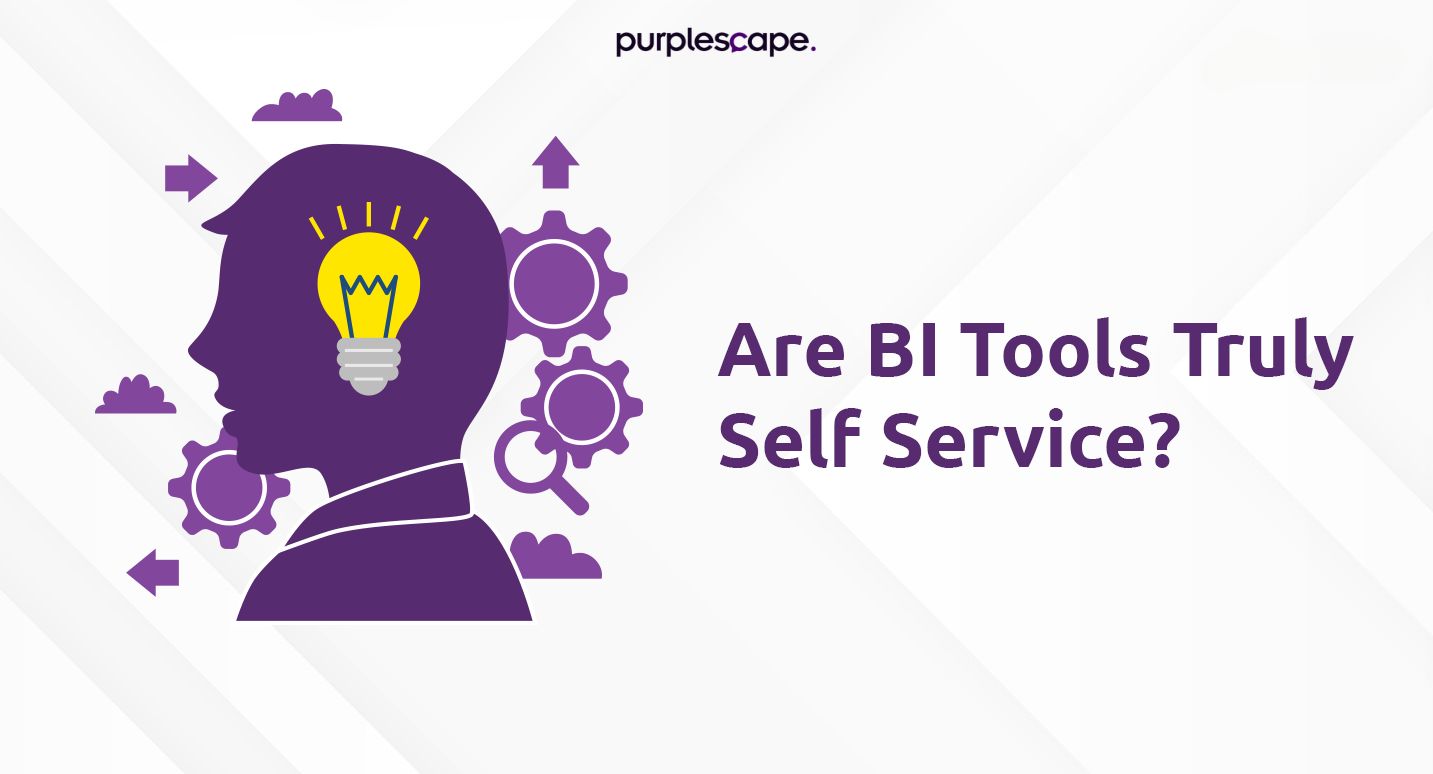
Introduction
Businesses are hoarding mountains of data. Data is collected from every resource over the Internet. But most companies are struggling to convert that data into actionable insights. In many cases, it’s because their analytics software is not user-friendly.
All your activities irrespective of whether you’re a marketing leader or a finance head, are dependent on data-driven insights. Data comes from a variety of sources like web analytics, internal sources, ERP tools, and more. The need to find the right insights, reports, and data to aid you in data-driven decision-making is huge.
Here we’ll look at modern self-service analytics, a paradox in itself that claims to be self-service, whereas reality is quite far from it. But before we delve deeper into analyzing the flaws of the subject, we need to understand what is self-serve analytics and how it differs from traditional BI tools.
A Tale of Two Business Intelligence Approaches
Business intelligence is a technology-driven process for analyzing data and presenting it in a format that is easy to understand and use.
Gartner defines self-service analytics as a form of business intelligence (BI) in which line-of-business professionals are enabled and encouraged to perform queries and generate reports on their own, with nominal IT support. Self-service analytics is often characterized by simple-to-use BI tools with basic analytic capabilities and an underlying data model that has been simplified or scaled down for ease of understanding and straightforward data access.
The main difference between business intelligence and self-service analytics is that business intelligence is dependent on internal IT support while self-service analytics allows users to access and analyze data on their own. The result in both cases is presented in a variety of formats, such as charts, graphs, and reports.
The difference between both can be easily explained with the process followed by the two approaches.
Traditional BI
- Business user comes out with the requirement for the report or dashboard
- The user submits his or her request to the IT department
- The IT team extracts the required data and then loads into it a warehouse for analysis
- Based on the user requirement, the IT team creates the data visualization
- Business user approves the report/dashboard or requests changes with the IT team
Self Service BI
- Business user approaches the IT team for the set of relevant data to build a report/dashboard
- IT team extracts, munges, and presents the data in the required format ready to load into a self-service tool
- Business users upload the data into the self-service tools and start querying to organize data for the report
- The business user builds the report or dashboard as per requirement using a simple point-and-click action
As you can see the responsibility matrix shifts to the business user’s end in the case of self-serve analytics tools. However, has it happened based on the intent is something that’s waiting to be seen.
The Story Behind Modern Self Service Tools
The current adoption rate of Business Intelligence tools is as low as 35% – Gartner
The need for self-service analytics was born out of a noble intention. Reduce the dependencies on internal IT departments to pull data insights and empower end users to create customized data visualizations with point and click GUIs.
Simply, Power Back to the People.
Alas! The current state of organizations investing in self-service analytics tools has a different story to tell. The process of creating dashboards has become so much convoluted that each of these organizations is forced to invest in specialized teams just to derive insights.
In the words of one of the most revered DC characters, Harvey Dent
Right from technological skill requirements to scalability, there are potential problems with self-service Business Intelligence (BI) which we will investigate further in this blog.
Top 3 Areas Current Self-Service BI Tools Need to Address
We have so far spoken about how self-service analytics is different from traditional BI and the story of its inception. But the lingering question and the topic of this blog is, are self-service tools truly “self-service”? This is where our thought process takes a different route, as the existing self-service tools have the following top problem areas that don’t deem them as self-service.
Ease of Use
Even though modern day self-service BI tools started out to reduce external dependencies, the intent digressed into an undesirable format. Currently, these tools demand a certain degree of expertise that requires training, certifications, and more. The complexity of operating these tools exponentially increases with the amount of data being collected and processed. It inevitably ends in the hands of the IT department to sort through and produce relevant dashboards or reports.
A few organizations have tried to work around this problem by including top business users in IT or the other way around. Introducing IT professionals to business units or functions depending on the size, but at the end of the day, having this approach defeats the purpose of being self-service.
Additional Overheads
As explained previously, some of the modern-day tools demand specialized skillsets to create reports and dashboards. Some organizations have gone ahead and set up a team of data engineers. In cases of large enterprises with access to strong budgets, they have even gone to the length of creating specialized business units to handle the data practices of various functions.
This all boils down to overheads in terms of compensation and benefits which adds up to the huge license fee shelled out to use these products.
Time Loss
Most self-service analytics tools take months and years to master. Even then for a seasoned user to create dashboards and reports, it will take him or her a specific amount of time. For momentary information needs the current suite of self-service tools is not sufficient.
Now to add to the woe, most of these tools create insights based on historical data. This implies for every weekly, fortnightly, or monthly meeting needs a fresh set of dashboards or reports to be produced. This will add to the already existing time delay which seriously hinders the decision-making process for business leaders.
The Way Forward for Self Serve Analytics is Conversational
Gartner believes that moving forward, the dashboards will be replaced with automated, conversational, mobile, and dynamically generated insights customized to a user’s needs and delivered to their point of consumption.
For self-serve data analytics to be true “self-service”, it has to center around the user and how convenient it is to access insights. In layman’s terms, modern day self-service BI tools need to let you as an end user Talk to your Data®
Only then can data access be simplified, and users get customized visualization capabilities.
Is there a way for end users to converse with their data, and derive insights for the momentary data questions they have? Specifically, in the language, they speak?
Meet Kea – Our Smart Virtual Data Assistant. Kea tries to simplify data access and bring clarity via a simple conversational interface. Train Kea on datasets of any size and variety to get straight answers to your questions on data, without the need to sift through complex reports, dashboards, and metrics. See Kea in action intuitively pick out the best way to visualize data and present it to you as an end user.